Intelligent agents, as a key technology in artificial intelligence (AI), have become central to a wide range of applications in both scientific research and business operations. These autonomous entities, designed to perceive their environment and adapt their behavior to achieve specific goals, are reshaping industries and driving innovation. This post provides a detailed analysis of the current state of intelligent agents, including definitions, theoretical and practical perspectives, technical characteristics, examples of business applications, and future prospects.
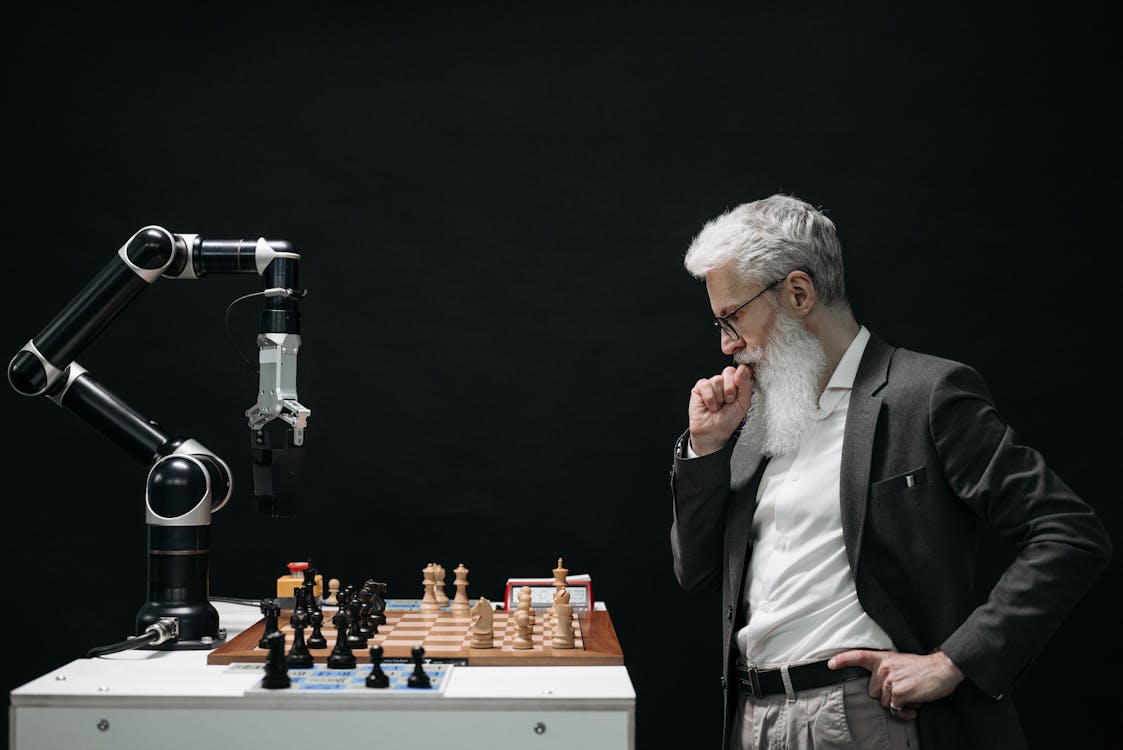
Definitions and Terminology
Intelligent agents are broadly defined as autonomous systems that can perceive and interact with their environments using sensors and actuators. Their autonomy enables them to make decisions and execute actions without constant human intervention. They operate with a specific goal or objective, which guides their decision-making processes. These entities may exist in either physical or virtual environments, ranging from robots in manufacturing plants to algorithms in financial markets.
A critical aspect of intelligent agents is their ability to reason about changes in their environments. For example, a self-driving car must detect obstacles, predict the behavior of other vehicles, and make decisions in real time to ensure safety. Multi-agent systems, a subset of intelligent agent technologies, involve multiple agents working collaboratively or competitively, depending on the system’s design.
Theoretical and Practical Perspectives
From a theoretical perspective, intelligent agents are grounded in computational intelligence, agent-based modeling, and systems theory. Computational intelligence incorporates methods like neural networks, evolutionary computation, and fuzzy logic to enable agents to process information, make decisions, and adapt to new situations. Agent-based modeling (ABM) is another crucial framework, allowing researchers to simulate complex systems by representing individual components as agents.
Practically, intelligent agents are applied in domains requiring automation, optimization, and real-time decision-making. Examples include recommendation systems in e-commerce, autonomous vehicles, and virtual assistants. These systems employ agent-based principles to enhance customer experiences, optimize resource allocation, and improve efficiency.
Technical Characteristics and Supporting Technologies
Intelligent agents have three primary characteristics: perception, reasoning, and action. Perception involves using sensors to gather data from the environment. Reasoning enables agents to analyze data and make decisions, often using machine learning or rule-based systems. Finally, action is carried out through actuators or communication mechanisms.
Supporting technologies include machine learning, natural language processing (NLP), and cloud computing. Machine learning allows agents to improve through experience, NLP enables effective communication with users, and cloud computing provides the infrastructure necessary for scalable agent-based systems. Additionally, the Internet of Things (IoT) expands the scope of intelligent agents by integrating them with physical devices.
Examples of Intelligent Agents in Business
Intelligent agents are widely used in business to enhance operations and decision-making. In e-commerce, platforms like Amazon use intelligent agents to recommend products based on user preferences and behavior, driving engagement and sales. Chatbots in customer service automate responses to frequently asked questions, improving efficiency and customer satisfaction.
In the transportation sector, intelligent agents are central to the development of autonomous vehicles. These agents navigate, detect obstacles, and communicate with other vehicles to ensure safety. Similarly, logistics companies use intelligent agents to optimize delivery routes, reducing costs and improving reliability. In smart cities, agents manage traffic systems, adjust signal timings, and minimize congestion to create more efficient urban environments.
Future Prospects for Businesses and Science
The future of intelligent agents is closely tied to advancements in emerging technologies such as quantum computing and advanced robotics. Quantum computing will enable agents to process massive amounts of data at unprecedented speeds, while robotics will enhance their ability to perform physical tasks. These developments will open new opportunities in finance, healthcare, logistics, and beyond.
In science, intelligent agents will continue to play a pivotal role in modeling complex systems, such as disease spread, climate change, and human cognition. Their adaptability and autonomy make them indispensable in addressing global challenges. Businesses will increasingly rely on intelligent agents for predictive analytics, decision support, and automation, enabling greater efficiency and innovation.
Conclusion
Intelligent agents are a transformative technology that is revolutionizing industries and scientific research. Their ability to operate autonomously, adapt to dynamic environments, and collaborate with other systems makes them essential in addressing complex challenges. As technology continues to evolve, intelligent agents will drive innovation, improve efficiency, and contribute to sustainable development in business and science.